Automated Decision Making: the role of meaningful human reviews
12 Apr 2019 03:15 PM
In the first detailed element of our AI framework blog series, Reuben Binns, our Research Fellow in AI, and Valeria Gallo, Technology Policy Adviser, explore how organisations can ensure ‘meaningful’ human involvement to make sure AI decisions are not classified as solely automated by mistake.
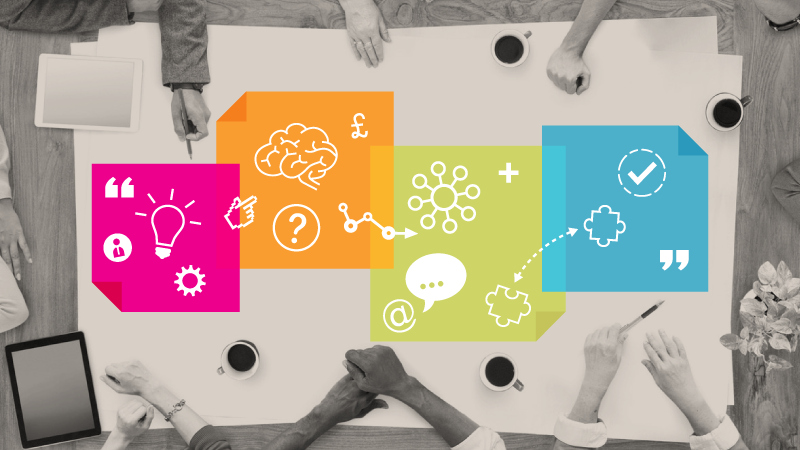
This blog forms part of our ongoing work on developing a framework for auditing AI. We are keen to hear your views in the comments below or you can email us.
Artificial Intelligence (AI) systems[1] often process personal data to either support or make a decision. For example, AI could be used to approve or reject a financial loan automatically, or support recruitment teams to identify interview candidates by ranking job applications.
Article 22 of the General Data Protection Regulation (GDPR) establishes very strict conditions in relation to AI systems that make solely automated decisions, ie without human input, with legal or similarly significant effects about individuals. AI systems that only support or enhance human decision-making are not subject to these conditions. However, a decision will not fall outside the scope of Article 22 just because a human has ‘rubber-stamped’ it: human input needs to be ‘meaningful’.
The degree and quality of human review and intervention before a final decision is made about an individual is the key factor in determining whether an AI system is solely or non-solely automated.
Board members, data scientists, business owners, and oversight functions, among others, will be expected to play an active role in ensuring that AI applications are designed, built, and used as intended.
The meaningfulness of human review in non-solely automated AI applications and the management of the risks associated with it are key areas of focus for our proposed AI Auditing Framework and what we will be exploring further in this blog.
What’s already been said?
Both the ICO and the European Data Protection Board (EDPB) have already published guidance relating to these issues. The key messages are:
- Human reviewers must be involved in checking the system’s recommendation and should not “routinely” apply the automated recommendation to an individual;
- reviewers’ involvement must be active and not just a token gesture. They should have actual “meaningful” influence on the decision, including the “authority and competence” to go against the recommendation; and
- reviewers must ‘weigh-up’ and ‘interpret’ the recommendation, consider all available input data, and also take into account other additional factors’.
Are there additional risk factors in complex systems?
The meaningfulness of human input must be considered in any automated decision-making systems however basic (e.g. simple decision trees). In more complex AI systems however, we think there are two additional factors that could potentially cause a system to be considered solely-automated. They are:
- Automation bias
- Lack of interpretability
Click here for the full blog post